在评估多分类模型性能时,我们经常会使用一些指标来衡量其表现。其中,micro-averaging、macro-averaging 和 weighted-averaging 是常见的评估指标之一。它们在衡量分类器的精确度、召回率和 F1 分数时发挥着重要作用。
假设:三分类的真实标签和预测标签如下:
y_true = ['好评', '好评', '好评', '中评', '中评', '差评', '差评', '差评', '差评', '差评'] y_pred = ['好评', '好评', '好评', '好评', '中评', '差评', '好评', '中评', '差评', '中评']
对应的混淆矩阵:
好评 中评 差评 好评 3 0 0 中评 1 1 0 差评 1 2 2
每个类别的评估分数:
精确率: [0.6 0.33333333 1. ] 召回率: [1. 0.5 0.4 ] f1-score: [0.75 0.4 0.57142857]
from sklearn.metrics import precision_score from sklearn.metrics import recall_score from sklearn.metrics import f1_score from sklearn.metrics import confusion_matrix import pandas as pd if __name__ == '__main__': y_true = ['好评', '好评', '好评', '中评', '中评', '差评', '差评', '差评', '差评', '差评'] y_pred = ['好评', '好评', '好评', '好评', '中评', '差评', '好评', '中评', '差评', '中评'] # 混淆矩阵 labels = ['好评', '中评', '差评'] matrix = confusion_matrix(y_true, y_pred, labels=labels) matrix = pd.DataFrame(matrix, columns=labels, index=labels) print(matrix) print() # 返回所有类别分数 result = precision_score(y_true, y_pred, average=None, labels=['好评', '中评', '差评']) print('精确率:\t\t', result) result = recall_score(y_true, y_pred, average=None, labels=['好评', '中评', '差评']) print('召回率:\t\t', result) result = f1_score(y_true, y_pred, average=None, labels=['好评', '中评', '差评']) print('f1-score:\t', result)
1. micro-averaging
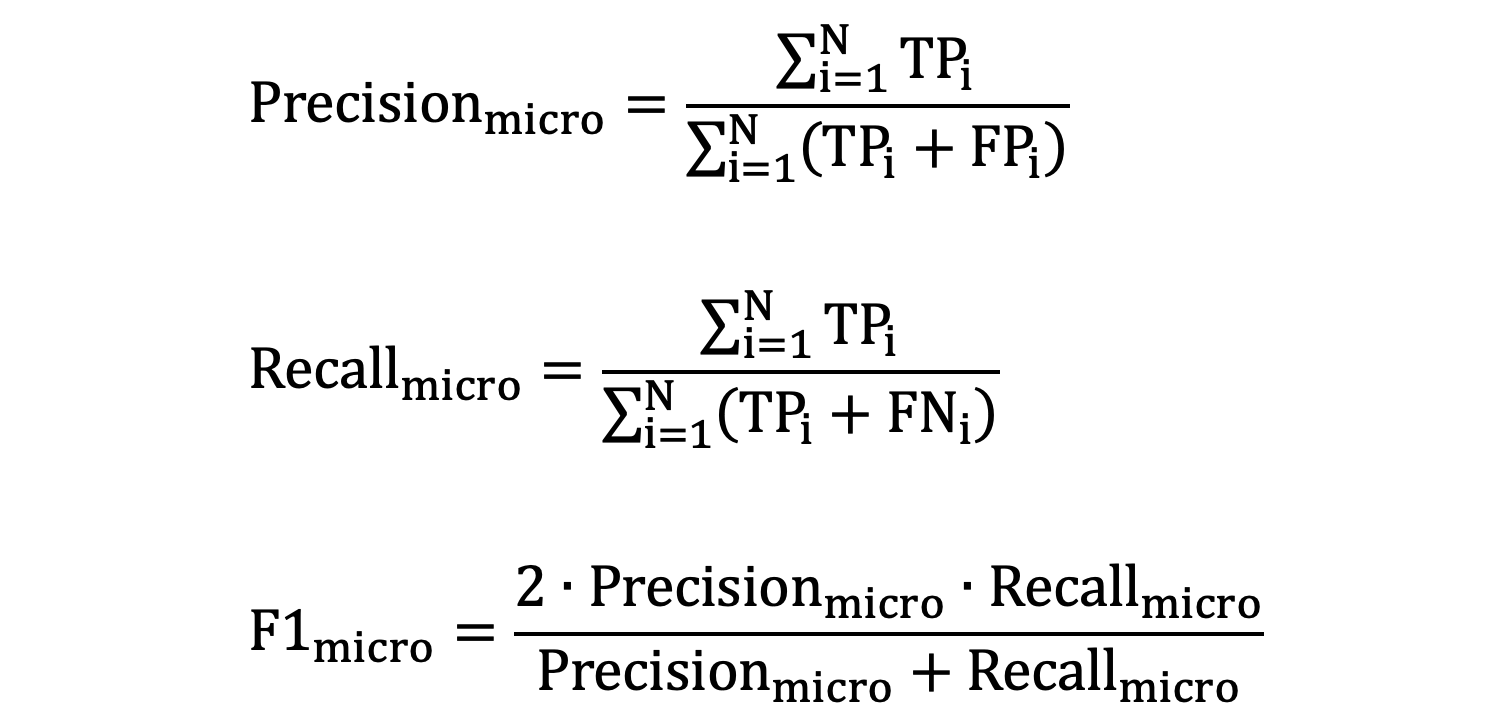
- \(N\) 是类别总数
- \(TP_{i}\) 是第 𝑖 个类别的 TP 之和
- \(FP_{i}\) 是第 𝑖 个类别的 FP 之和
- \(FN_{i}\)是第 𝑖 个类别的 FN 之和
我们以前面例子为例:
好评: TP = 3 (预测为好评且实际为好评) FP = 1+1 = 2 (预测为好评但实际为中评和差评) TN = 1+2+2 = 5 (预测不是好评且实际也不是好评,包括中评和差评) FN = 0+0 = 0 (实际为好评但预测不是好评) 中评: TP = 1 (预测为中评且实际为中评) FP = 0+2 = 2 (预测为中评但实际为好评和差评) TN = 3+2+1+0 = 6 (预测不是中评且实际也不是中评,包括好评和差评) FN = 1+0 = 1 (实际为中评但预测不是中评) 差评: TP = 2 (预测为差评且实际为差评) FP = 0+0 = 0 (没有将其他类别预测为差评) TN = 3+1+1 = 5 (预测不是差评且实际也不是差评,包括好评和中评) FN = 1+2 = 3 (实际为差评但预测不是差评) 每个类别的 TP、FP、TN、FN 值分别是: 好评:TP=3, FP=2, TN=5, FN=0 中评:TP=1, FP=2, TN=6, FN=1 差评:TP=2, FP=0, TN=6, FN=3 此时: TP = 3 + 1 + 2 = 6 FP = 2 + 2 + 0 = 4 TN = 5 + 7 + 6 = 18 FN = 0 + 1 + 3 = 4
使用上述计算得到的 TP、FP、TN、FN 值:
- micro-averaged precision:
TP/(TP+FP) = 6/(6+4) = 0.6
- micro-averaged recall:
TP/(TP+FN) = 6/(6+4) = 0.6
- micro-averaged f1-score:
2*(micro-averaged precision * micro-averaged recall) / (micro-averaged precision+micro-averaged recall) = 2*(0.6*0.6)/(0.6+0.6) = 0.6
from sklearn.metrics import precision_score from sklearn.metrics import recall_score from sklearn.metrics import f1_score if __name__ == '__main__': y_true = ['好评', '好评', '好评', '中评', '中评', '差评', '差评', '差评', '差评', '差评'] y_pred = ['好评', '好评', '好评', '好评', '中评', '差评', '好评', '中评', '差评', '中评'] result = precision_score(y_true, y_pred, average='micro') print('精确率:\t\t', result) result = recall_score(y_true, y_pred, average='micro') print('召回率:\t\t', result) result = f1_score(y_true, y_pred, average='micro') print('f1-score:\t', result)
精确率: 0.6 召回率: 0.6 f1-score: 0.6
2. macro-averaging
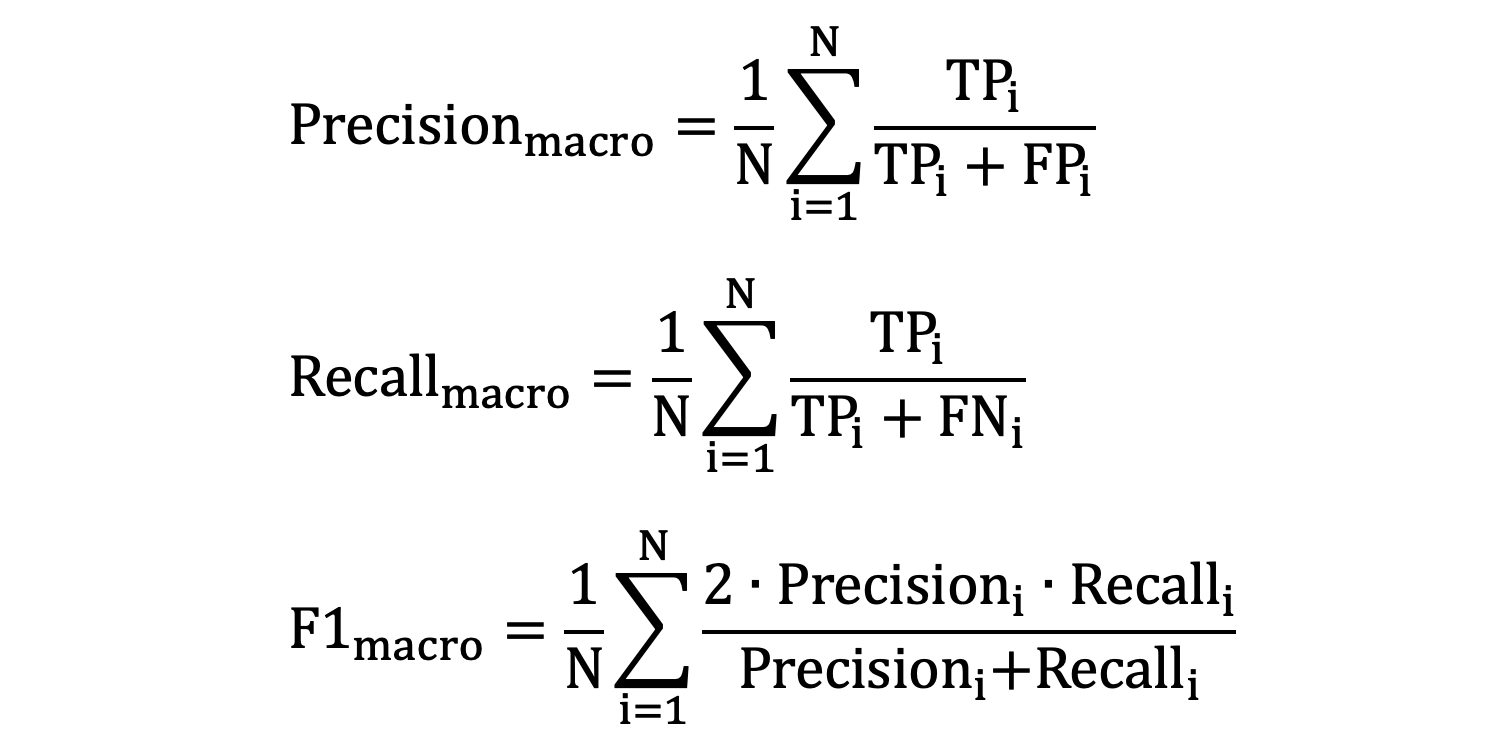
macro-averaging 计算每个类别的精确度、召回率和 F1 分数,然后对它们取算术平均值。
好评: 精确率:0.6 召回率:1.0 f1-score:0.75 中评: 精确率:0.33 召回率:0.5 f1-score:0.4 差评: 精确率:1 召回率:0.4 f1-score:0.57
- macro-averaged precision
:
(0.6 + 0.33 + 1) / 3 = 0.64 - macro-averaged recall:(1.0 + 0.5 + 0.4) / 3 = 0.63
- macro-averaged f1-score:(0.75 + 0.4 + 0.57) / 3 = 0.57
from sklearn.metrics import precision_score from sklearn.metrics import recall_score from sklearn.metrics import f1_score if __name__ == '__main__': y_true = ['好评', '好评', '好评', '中评', '中评', '差评', '差评', '差评', '差评', '差评'] y_pred = ['好评', '好评', '好评', '好评', '中评', '差评', '好评', '中评', '差评', '中评'] result = precision_score(y_true, y_pred, average='macro') print('精确率:\t\t', result) result = recall_score(y_true, y_pred, average='macro') print('召回率:\t\t', result) result = f1_score(y_true, y_pred, average='macro') print('f1-score:\t', result)
精确率: 0.6444444444444445 召回率: 0.6333333333333333 f1-score: 0.5738095238095239
3. weighted-averaging
假设:共有 100 个样本:
好评:70,精度为:0.3
中评:20,精度为:0.6
差评:10,精度为:0.9
宏平均 = (0.3 + 0.6 + 0.9) / 3 = 0.6
在大多数样本上,模型只有 0.3 的精度,但是宏平均的精度却达到了 0.6。所以,宏平均不能真实的反映出模型在大多数样本上的表现。
weighted-averaging 计算每个类别的精确度、召回率和 F1 分数,然后将它们乘以每个类别的样本数或权重,最后将所有类别的加权平均值。它能够反应出样本在大多数样本上的表现。
加权平均:0.3 * 0.7 + 0.6 * 0.2 + 0.9 * 0.1 = 0.42,由此可以看到加权平均更加能够反映出模型在大多数样本上的表现。
接着前面的例子:
由 y_true = ['好评', '好评', '好评', '中评', '中评', '差评', '差评', '差评', '差评', '差评']
可知每个类别的权重分别为:0.3、0.2、0.5
- weighted-averaged precision:0.6 * 0.3 + 0.33 * 0.2 + 1 * 0.5 = 0.746
- weighted-averaged recall:1 * 0.3 + 0.5 * 0.2 + 0.4 * 0.5 = 0.6
- weighted-averaged f1-score:0.75 * 0.3 + 0.4 * 0.2 + 0.57 * 0.5 = 0.59
from sklearn.metrics import precision_score from sklearn.metrics import recall_score from sklearn.metrics import f1_score if __name__ == '__main__': y_true = ['好评', '好评', '好评', '中评', '中评', '差评', '差评', '差评', '差评', '差评'] y_pred = ['好评', '好评', '好评', '好评', '中评', '差评', '好评', '中评', '差评', '中评'] result = precision_score(y_true, y_pred, average='weighted') print('精确率:\t\t', result) result = recall_score(y_true, y_pred, average='weighted') print('召回率:\t\t', result) result = f1_score(y_true, y_pred, average='weighted') print('f1-score:\t', result)
精确率: 0.7466666666666667 召回率: 0.6 f1-score: 0.5907142857142857